by Harley G. Lavelle
1. Introduction
Artificial Intelligence (AI) algorithms and technologies are poised to, in the increasingly near future and present, embed themselves into many of the structures, institutions, and processes relevant to global justice, including those relevant to refugee claimants’ rights and welfare (Molnar and Gill 7-8). Several scholars have promoted the potential benefits that AI technologies may bring to asylum seekers and international human rights. AI can, for example, help civil managers choose host communities that increase the likelihood of successful refugee integration (Bansak et al. 325). However, other scholars claim AI is already doing harm to refugee claimants seeking entry into Canada, not to mention other countries outside the scope of this essay. This essay explores the overall impacts of AI on refugee claimants seeking entry to Canada and analyzes whether those impacts can be expected to be negative or positive. Although AI offers many potential benefits for diverse populations within society, including refugees and asylum seekers, its usage in Canada thus far damages their welfare and potentially infringes on their rights by (i) lacking necessary transparency and oversight to provide privacy protections for an already vulnerable population; and through (ii) discrimination, bias, and error potentially refusing to recognize refugee claimants as such and grant them corresponding rights and protections. However, optimistically, although the use of AI poses risks, AI retains the potential to contribute positively to refugee claimants’ rights and welfare in the future. Accordingly, this essay concludes with recommendations from scholars that, if adopted, would improve the use of AI in Canada’s refugee systems going forward.
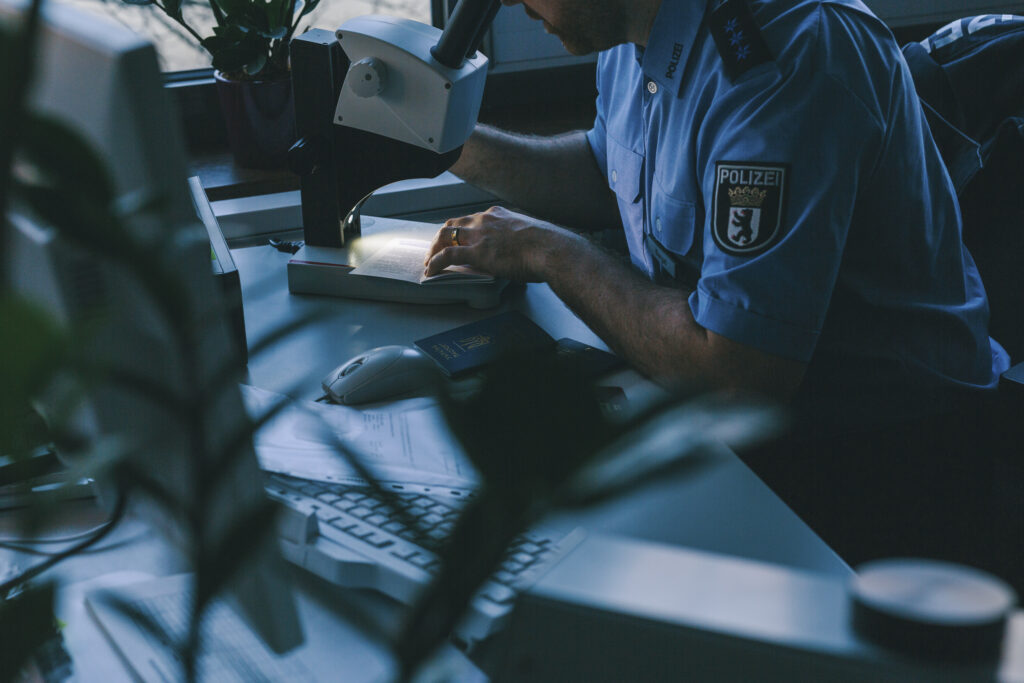
2. Benefits of AI Technologies for Refugees
Before continuing further, it is important to address the correct usage of the terms refugee, asylum seeker, and refugee claimant. Following the 1951 Geneva Convention, a refugee is someone who cannot return to their country of origin due to fears of persecution based on political opinion and other forms of discrimination (Moldovan 682). Additionally, an asylum seeker or refugee claimant is someone who is seeking the legal recognition of refugee status, although it has not yet been granted to them (Moldovan 683).
The limited academic literature that discusses the interaction of AI and refugee claimants and/or human rights and global justice more broadly are primarily optimistic. This literature generally looks toward the new technological abilities that AI will enable for refugee claimants and relevant policymakers. For example, as stated, an algorithm has been developed to place refugees in host communities that would maximize the success of their integration process (Bansak et al. 325). Manjikian noted that the success of the integration process is a cornerstone of the refugee experience in the host country and may enable refugees to become socially and politically engaged ( 51). Throughout the determination and integration processes, asylum seekers encounter a heightened sense of “in-betweenness” due to a lack of belonging to one place or another (Manjikian 50). Using AI to improve refugee integration could lessen this feeling of “in-betweenness”. Additionally, scholars promote the potential for AI to aid refugee claimants and international human rights by other, disparate means. Certain scientists have developed a model for predicting and simulating the movement of asylum-seeking groups, which might enable policymakers to better understand their movements and plan accordingly to support them (Suleimenova et al. 1). This could be beneficial to countries neighboring the country of origin of the asylum seekers, as well as Canada. For example, research by Kaida et al. found that it is likely for refugees to migrate from their initial point of settlement within the host country (6). The research by Kaida et al. and the model developed by Bansak et al. provide useful insight for proper and efficient resource allocation and meaningful refugee placement within the host country. These examples demonstrate only a hint of AI’s potential benefits as technologies that can be used by policymakers to support refugees during the integration and determination process.
3. Negative Effects of AI
However, to accurately assess the reality of AI’s impacts on refugees’ welfare and rights, it is necessary to remove rose-colored lenses. The optimism about how AI may enable new technologies for refugees and policymakers must be tempered with a sober examination of how AI may also negatively affect the welfare and rights of asylum seekers and refugees in Canada. As Rousseau et al. state, “refugee determination is one of the most complex adjudication functions in industrialized societies.” (43). Difficulties arise when parts of this already complex and difficult process are delegated to artificial intelligence. It is particularly concerning how the vast amounts of data gathered and analyzed by the AI systems will be protected, as well as what information the AI algorithms will be built upon and learning from.
Given that this field of academic study within human rights is in its nascent stages, particularly in Canada, I rely heavily on a report entitled “Bots at the Gate: A Human Rights Analysis of Automated Decision-Making in Canada’s Immigration and Refugee System,” authored by Petra Molnar and Lex Gill as a collaboration between The Citizen Lab and The University of Toronto Faculty of Law’s International Human Rights Program. This is the most recent major report to analyze concerns and offer recommendations for the use of AI and automated decision-making by the Canadian government in its immigration and refugee systems.
4. Data Privacy, Protections, and Transparency
In recent decades, the increasing use of technology, whether in managing the International Space Station or the subway for citizens’ daily commute, has caused a proliferation of digital data. Meanwhile, the privacy of data and the transparency around its usage has substantially diminished. This new reality also applies to refugee claimants. Vast amounts of data are collected regarding asylum seekers as they cross borders and encounter governments of other countries. However, the protection and use of said data are unclear to them and researchers. A United Nations (UN) audit report concluded that it is unclear if asylum seekers were informed of the use of their data by the UN, governments, or non-governmental bodies (Madianou 592). What is particularly worrisome about this is that Canada has entered into data-sharing agreements with foreign countries, and outsiders cannot know if the refugee claimant’s information may even be shared with the refugee claimant’s country of origin (Molnar and Gill 43). As Molnar and Gill suggest, refugee claimants are especially vulnerable to privacy concerns, in part because repressive countries of origin may “weaponize” this information against them (Molnar and Gill 43). This poses significant safety risks to asylum seekers in the event that their application is rejected and they are forced to return to their country of origin. Additionally, even if not intentionally shared, if this data is improperly secured, repressive countries of origin could gain access to sensitive data via breaches or cyberespionage and apply punitive measures to rejected asylum seekers.
Moreover, the code used for Canada’s AI and automated decision-making for refugee determination is not public and open-source (Molnar and Gill 2). This makes it particularly difficult for external actors to gain insight into it – or provide oversight for it. The Treasury Board of Canada Secretariat released a paper containing principles for the responsible use of AI in the Canadian government (Molnar and Gill 16). Unfortunately, the information in the paper is merely a recommendation and has no significant impact on the practice of AI use within the government. Due to the lack of transparency, independent oversight, and standards to govern the system, issues of data privacy and protection become more difficult to identify and rectify when they arise.
5. Discrimination, Bias, and Error
Most importantly, “the opaque nature of immigration and refugee decision-making creates an environment ripe for algorithmic discrimination” (Molnar and Gill 33). Issues arise in how decision-makers set the algorithms and baseline questions for an automated decision-making system to determine an applicant’s refugee status. AI analyzes and learns from data, some of which may potentially contain human biases, therefore legitimating any form of discrimination present in the training data (Madianou 590). For example, in 2017, the RCMP “without any clear rationale, and apparently on its own initiative” collected 5,000 questionnaires from asylum seekers using arguably Islamophobic, or at least Islam-focused, questions such as the individual’s perception of women who do not wear a hijab, their opinions on ISIS and the Taliban, as well as the number of times a day the individual prayed (Molnar and Gill 19). One can imagine the potential problems with an automated decision-making system that was created “without any clear rationale” and similarly-programmed, without oversight or transparency, to flag refugee applications based on how often the asylum seeker prayed. Embedding pre-existing refugee determination issues into a computer system that touches a larger portion of, or all, incoming refugee claimants dramatically increases the number of people affected and potentially discriminated against. To provide an understanding of the reach the AI system in Canada would have, in 2019, Immigration, Refugees, and Citizenship Canada and Canada Border Services Agency processed over 64,000 refugee claims (Statistics Canada). The automated process could apply a problematic methodology to all refugee cases it touches. This would lock in and mass distribute any problems that were in the training data when the algorithm was designed.
4. Conclusion – Remnants of Optimism
Canada’s application of AI and automated decision-making to refugee issues, including refugee determination, need not be dreaded as might be the impression left by the arguments above. AI has tremendous potential to positively impact many aspects of economic and social life for society, including diverse and vulnerable populations within society – that is to say, including refugees. However, to reassure the public that these impacts will be net positive, or at least not equaled by disconcerting negative potential effects, the Canadian government will have to make important changes. Those changes might begin most effectively by integrating the recommendations from Molnar and Gill to address the issues of transparency, data privacy and protection, and bias, discrimination, and error, discussed in this essay. Along with four other recommendations, Molnar and Gill recommend (1) the Canadian government regularly publish complete and detailed reports containing important disclosures regarding the AI and automated decision-making systems and their operation; (2) halt use of any such technologies until existing systems adhere to a legally binding government-wide Standard or Directive regarding the responsible use of these technologies; and (6) commit to making all relevant code public and open source by default, with limited exceptions only for reasons of privacy and national security (Molnar and Gill 63-66). Adopting all of Molnar and Gill’s recommendations, Canadians could be reassured that the use of AI and automated decision-making in refugee determination would be a net positive. However, until then, despite AI’s promise in the field and the optimism of some scholars, AI and automated decision-making in Canada’s refugee determination systems risk damaging refugee claimants’ welfare and potentially infringing on their rights through such a process’s lack of transparency, risks to data privacy and protection, and potential for discrimination, bias, and error.
Works Cited
“Asylum Claims by Year.”Statistics Canada, 2020, www.canada.ca/en/immigration-refugees-citizenship/services/refugees/asylum-claims/asylum-claims-2019.html.
Bansak, Kirk, et al. “Improving Refugee Integration through Data-Driven Algorithmic Assignment.” Science, vol. 359, no. 6373, 19 Jan. 2018, p. 325, DOI:10.1126/science.aao4408.
Kaida, Lisa, et al. Are Refugees More Likely to Leave Initial Destinations than Economic Immigrants? Cat. No. 11F0019M, No. 411. Statistics Canada, 2020, pp. 5-23., www150.statcan.gc.ca/n1/pub/11f0019m/11f0019m2020004-eng.pdf.
Madianou, Mirca. “The Biometric Assemblage: Surveillance, Experimentation, Profit, and the Measuring of Refugee Bodies.” Television & New Media, vol. 20, no. 6, 2019, pp. 581–599, DOI:10.1177/1527476419857682.
Manjikian, Lalai. “Refugee ‘in-betweenness’: a proactive existence.” Refuge, vol. 27, no. 1, 2010, pp. 50-58. Gale OneFile: CPI.Q, link-gale-com.ezproxy.lib.ryerson.ca/apps/doc/A269028061/CPI?u=rpu_main&sid=CPI&xid=d71a8e40.
Moldovan, Carmen. “The Notion Of Refugee. Definition And Distinctions.” CES Working Papers, vol. 8, no. 4, 2016, pp. 681-688, ezproxy.lib.ryerson.ca/login?url=https://search-proquest-com.ezproxy.lib.ryerson.ca/docview/1863562383?accountid=13631.
Molnar, Petra, and Gill, Lex. Bots at the Gate: A Human Rights Analysis of Automated Decision-Making in Canada’s Immigration and Refugee System. University of Toronto, the Citizen Lab, 2018, citizenlab.ca/wp-content/uploads/2018/09/IHRP-Automated-Systems-Report-Web-V2.pdf.
Rousseau, Cécile, et al. “The Complexity of Determining Refugeehood: A Multidisciplinary Analysis of the Decision-Making Process of the Canadian Immigration and Refugee Board.” Journal of Refugee Studies, vol. 15, no. 1, 2002, p. 43, DOI:10.1093/jrs/15.1.43.
Suleimenova, Diana, et al. “A Generalized Simulation Development Approach for Predicting Refugee Destinations.” Scientific Reports (Nature Publisher Group), vol. 7, 2017, p. 1, ProQuest, DOI:10.1038/s41598-017-13828-9.